Understanding the Concept of QH% PQQ A Deep Dive into Modern Data Management
In the ever-evolving landscape of digital technology, data management has become a central pillar for organizations striving for efficiency and insight. Among the various methodologies and terminologies that have emerged, one such concept worth exploring is QH% PQQ. Though it may sound obscure to the uninitiated, a comprehensive understanding of what QH% PQQ entails can illuminate the ways in which organizations can enhance their data workflows and decision-making processes.
What is QH% PQQ?
While QH% PQQ may not be a universally recognized term in the field of data management, it can be interpreted through its components QH%, standing for Quality Heuristic Percentage, and PQQ, meaning Process Quality Quotient. Together, these two concepts provide a foundation for assessing and improving the quality of data within organizations.
1. Quality Heuristic Percentage (QH%)
Quality Heuristic Percentage refers to a metric that evaluates the qualitative aspects of data. In data management, quality is paramount; poor-quality data can lead to misguided decisions, wasted resources, and diminished trust in analytics. QH% focuses on defining what constitutes quality within a specific context, including completeness, accuracy, reliability, and relevance.
Organizations can utilize QH% by setting clear benchmarks for each quality dimension. For example, if a company is managing customer data, it might decide that accurate contact information and up-to-date purchase history are crucial indicators of quality. By measuring how much of the data meets these criteria, businesses can establish a QH% score that reflects their current data integrity.
2. Process Quality Quotient (PQQ)
The Process Quality Quotient, or PQQ, complements the QH% by assessing the effectiveness of the processes involved in data collection, storage, and analysis. This metric evaluates how well an organization has designed its processes to ensure high-quality data outputs. Effective processes are essential for maintaining the integrity and quality of data as it moves through various stages of the data lifecycle.
To analyze PQQ, organizations can look at the systems and methodologies in place for data management. This could involve evaluating automated data entry systems, data cleaning procedures, and the protocols for data validation. By identifying bottlenecks or weaknesses in these processes, businesses can implement enhancements that improve both efficiency and the quality of the resulting data.
The Interplay Between QH% and PQQ
qh pqq
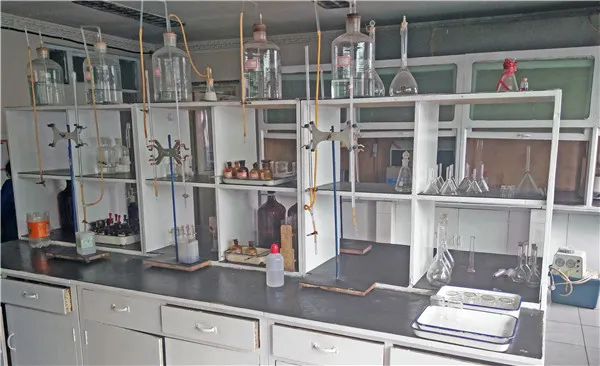
Understanding QH% and PQQ in isolation is important, but recognizing their interrelation is crucial for effective data management. High QH% cannot be achieved if the underlying processes (PQQ) are flawed. Conversely, even well-designed processes cannot ensure quality outputs if the foundational data is inadequately managed.
For instance, if a company employs an efficient data collection process but fails to validate the accuracy of incoming data, the QH% will inevitably suffer. On the other hand, if an organization emphasizes data accuracy through extensive validation checks but has outdated or poorly defined collection processes, discrepancies may still arise, leading to a suboptimal PQQ.
Implementing QH% PQQ in Organizations
Embarking on a journey to incorporate QH% and PQQ into data management involves several actionable steps
1. Assessment Begin with a thorough assessment of existing data quality and processes within the organization. Utilize metrics and KPIs to gauge current QH% and PQQ.
2. Goal Setting Define specific goals for both data quality and process efficiency. These goals should be measurable and aligned with the organization's broader objectives.
3. Continuous Improvement Implement a continuous feedback loop where data quality and process efficiencies are regularly evaluated and refined based on real-time insights and changing organizational needs.
4. Training and Culture Foster a culture that values data quality by providing training and resources for employees. Empower teams to understand the importance of QH% and PQQ in their daily operations.
5. Technology Integration Make use of modern technologies such as AI and machine learning to automate data quality assessments and streamline processes.
Conclusion
As organizations grapple with vast amounts of data daily, the importance of frameworks like QH% and PQQ cannot be overstated. By focusing on the quality of data and the efficacy of processes, businesses can build a strong foundation for insightful decision-making and sustainable growth. Embracing these concepts will not only improve operational efficiency but also foster trust in data-driven decisions, paving the way for future innovation and success.