Understanding PQ and Q A Deep Dive into Probability and Quality
In the fields of statistics and quality management, the terminology pqq and q often surfaces in discussions regarding probability and quality assurance. To grasp their significance, we need to understand the concepts of probability, quality metrics, and how they interrelate in practical applications—is it in manufacturing, services, or product development.
Probability, represented by p, plays a crucial role in assessing uncertainties. It provides a mathematical framework to quantify the likelihood of events occurring. For instance, when a factory is producing thousands of widgets, understanding the probability of defects in those widgets becomes essential. By analyzing historical data, manufacturers can establish their p-value, which represents the proportion of faulty items, thus enabling them to make informed decisions when it comes to improvements in their processes.
On the other hand, q often symbolizes a quality metric and is frequently used to denote the proportion of good products in a statistical sample. Quality assurance processes depend heavily on these metrics to ensure that products meet predefined standards before reaching the market. If we take the previous example of widgets, if 95% of them pass inspection, then the q-value would be 0.95. Here, the higher the q-value, the better the quality of the production line.
The relationship between p (probability of a defect) and q (quality metric) can be succinctly represented by the equation
\[ q = 1 - p \]
pqq q
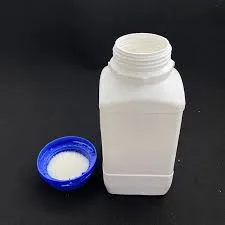
This means that if a product has a 5% defect rate (p = 0.05), it can be argued that the quality level (q) is 95%—a reassuring figure for both manufacturers and consumers alike. However, grasping this relationship is not merely an academic exercise; it has real-world implications in production and quality management.
Moreover, leveraging these metrics for continuous improvement requires adopting methodologies such as Six Sigma or Total Quality Management (TQM). In Six Sigma processes, organizations strive to reduce variability, thereby improving q while simultaneously decreasing p. Applying statistical methods and tools, teams can analyze data to identify sources of defects and implement corrective measures, ultimately pushing the probabilities in favor of quality improvements.
In service industries, p and q can similarly transform customer experiences. For instance, a customer support center may keep track of resolution rates and wait times. Here, p could represent the probability of a customer not receiving timely support, while q would relate to satisfactory service delivery. By systematically analyzing these metrics, businesses can enhance their service quality and build stronger customer loyalty.
Despite their theoretical nature, the concepts of p and q are easily applicable across various sectors of industries. The integration of technology—like data analytics and machine learning—into quality management ignites substantial improvements. Using predictive analytics, organizations can better forecast potential defects and systematically reduce p, which would consequently enhance q.
Finally, the constant monitoring of p and q is essential for sustainable success. By establishing feedback loops and utilizing tools like control charts, managers can keep tabs on performance and implement timely interventions. In doing so, they not only fulfill customer expectations but also foster a culture of excellence.
In conclusion, understanding the relationship between p (probability of defects) and q (quality metric) is fundamental for organizations striving for high-quality outcomes. This interplay drives continuous improvement and operational excellence across varied industries. As sectors evolve, maintaining a keen focus on these metrics will be crucial for sustaining competitive advantages and ensuring long-term viability in today's dynamic economy.